Generative AI was supposed to revolutionize content analysis. But recent studies reveal a different story. Can Google Gemini and other AI models truly deliver?
THE BIG PICTURE: Generative AI, hailed as a game-changer, struggles with complex analysis tasks. Two groundbreaking studies reveal these limitations. The hype around Google Gemini? It might be overblown.
Let’s dive into the details. One study examined Gen AI’s ability to understand long texts. The results were surprising. For a 520-page book, Google Gemini 1.5 Pro answered true/false questions correctly only 46.7% of the time. Gemini Flash? A mere 20%.
Study Findings at a Glance:
Model | Accuracy (True/False) |
---|---|
Gemini 1.5 Pro | 46.7% |
Gemini Flash | 20% |
GPT-4 | 55.8% |
These figures are startling. AI models, including Google’s latest, are falling short. Especially when tasked with long-form text analysis.
But that’s not all. Another study focused on vision language models (VLMs). These models also struggle with large visual contexts. Gemini 1.5 Flash, for instance, can process immense amounts of data quickly. Yet, it fails to grasp long-form context.
AI Capabilities Claimed by Google:
Task | Capacity |
---|---|
Video Analysis | 1 hour |
Audio Processing | 11 hours |
Text Analysis | 700,000+ words per query |
In a demonstration, Google showcased Gemini’s ability to analyze a 14-minute video in one minute. Impressive? Not quite. According to Marzena Karpinska, a postdoc at UMass Amherst and study co-author, these models don’t genuinely understand the content.
Karpinska’s team, including researchers from the Allen Institute for AI and Princeton, evaluated the AI’s performance. They posed true/false questions about recent fiction books, focusing on specific details and plot points. The results were disappointing.
More Study Results:
Model | Accuracy (True/False) |
---|---|
Gemini 1.5 Pro | 46.7% |
Gemini Flash | 20% |
GPT-4 | 55.8% |
GPT-4 achieved the highest accuracy at 55.8% on the NoCha (Novel Challenge) dataset. However, even accurate responses were marred by incorrect model-generated explanations.
“We’ve noticed that models struggle more with claims requiring consideration of larger portions of the book or the entire book,” said Karpinska. “They also have trouble with implicit information clear to human readers but not explicitly stated.”
The second study’s findings were equally troubling. It evaluated VLMs on tasks like mathematical reasoning, visual question answering (VQA), and character recognition. These models struggled as the visual context length increased.
VLM Study Findings:
Task | Performance |
---|---|
Transcribing handwritten digits | ~50% accuracy (6 digits) |
30% accuracy (8 digits) |
Michael Saxon, a PhD student at UC Santa Barbara and study co-author, noted that even basic reasoning tasks, such as recognizing and reading numbers, posed significant challenges. “That small amount of reasoning – recognizing a number and reading it – might be what is breaking the model,” he said.
These revelations raise serious questions about the real capabilities of generative AI. As companies integrate AI into their operations, they must be aware of these limitations.
Generative AI’s potential is vast, but so are its limitations. As we continue to explore these technologies, it’s crucial to maintain realistic expectations and stay informed about their actual capabilities.
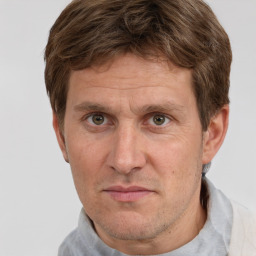
Immanuel Matthew is an experienced journalist with a passion for delivering accurate and timely news updates. As a seasoned professional at dynews.net, Immanuel Matthew ensures that every story meets the highest standards of journalistic integrity and clarity. Matthew brings insightful reporting to readers, covering a wide range of topics with depth and precision.